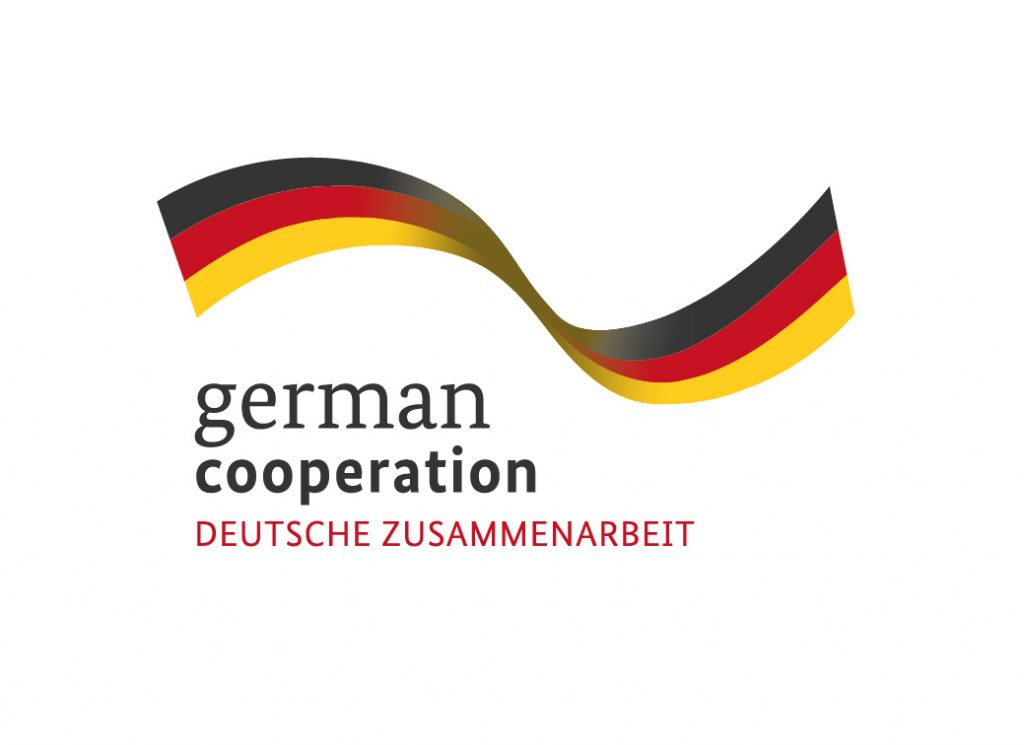
Earth Observation
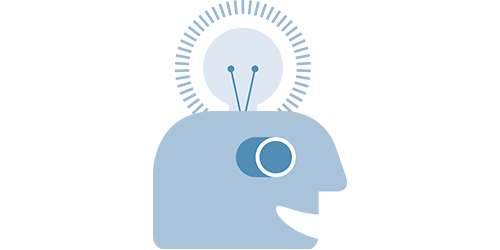
What is the opportunity for collaboration?
Through a co-creative process with key stakeholders, the ii2030 challenge aims to identify a sustainable model to improve and ensure open access to high-quality Earth observation data for use with machine learning (ML) applications. Rather than creating another tech solution, the process focuses on a strategic collaboration method between stakeholders to co-create effective change.
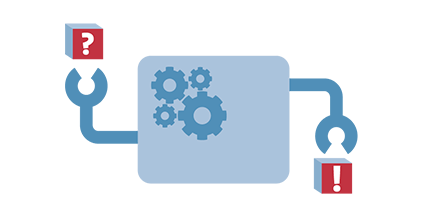
Why is this relevant?
Earth observation data can help to advance the Sustainable Development Goals (SDGs). More concretely, remote sensing data provide relevant information on soil, water, plant growth, pest, disease, weather-related damages, and climate impacts, to list a few examples. Access to such data can, for example, help farmers manage their farms more effectively, contributing to food security. These applications require high-quality “ground truth” data to train and validate the ML algorithms.
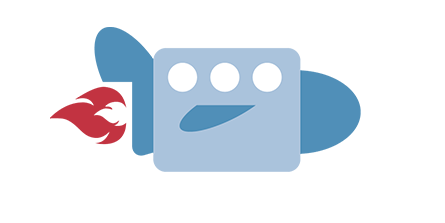
Which challenges are we addressing?
Radiant Earth Foundation has already created an open library called “Radiant MLHub,” which aggregates data, models, and standards for ML applications on Earth observation data. The library encourages widespread data collaboration, allowing users to access, store, register, and share datasets and models. Radiant ensures that the data is easily accessible and represents different geographies, leading practitioners to build better and more accurate models.
However, getting access to quality datasets and maintaining the library requires resources, which, thus far, have been generated through grants and other types of philanthropic support. The ii2030 process will identify sustainable ways for widespread data collaboration for Radiant, and other players in the ecosystem.
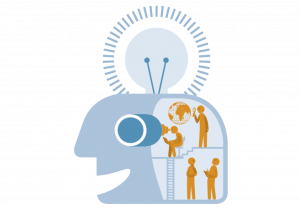
Who attended
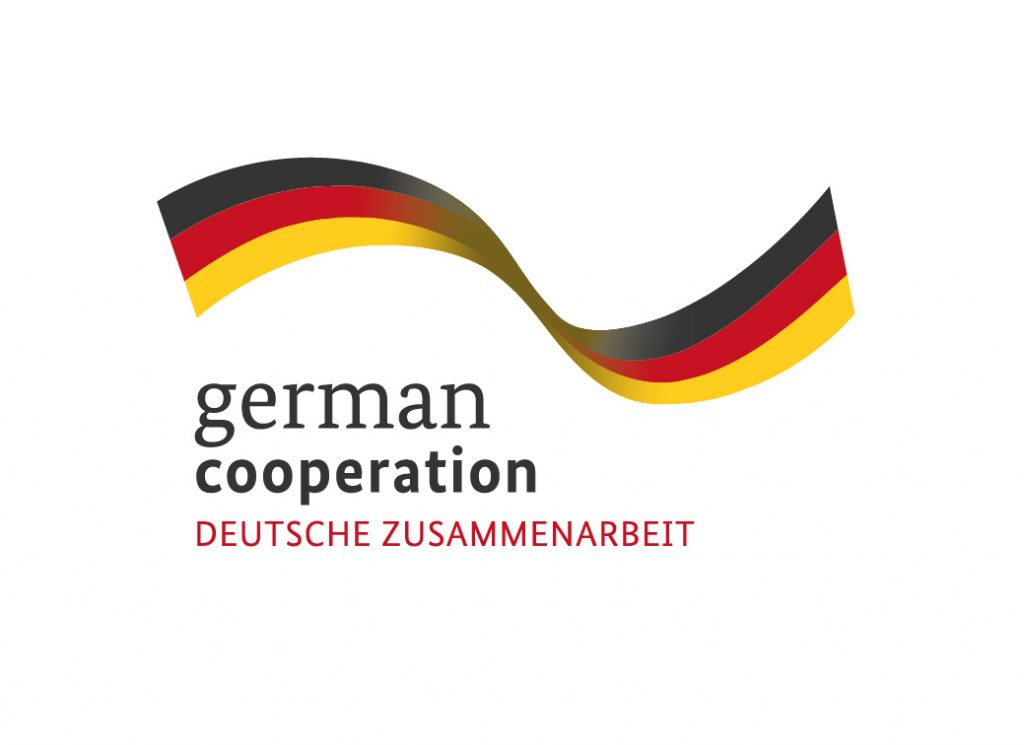




